In the realm of financial accounting and collections management, effective clearing control is essential to ensure accurate and streamlined processes. SAP FI-CA (Financial Contract Accounting) and FS-CD (Insurance Collections and Disbursements) are powerful modules that facilitate billing, collections, and payment processing for various industries particularly dealing with large volumes of payment transactions. Clearing open items has traditionally relied on rule-based algorithms, which might not always handle complicated cases and large amounts of data quickly. However, with the development of machine learning (ML) technology, these SAP modules can now make use of sophisticated algorithms to improve clearing control operations. As a consequence, accuracy is improved, manual labor is minimized, and decision-making is improved. Using machine learning, the business and the system can learn simultaneously. The ML application can start making clearing proposals for businesses to review and approve in the live environment. Once the business gains confidence level, it can let the application handle clearing automatically.
Clearing control is a fundamental aspect of SAP FI-CA/FS-CD that ensures the accurate allocation of incoming payments to outstanding invoices or accounts. It involves matching payments with open items based on predefined criteria, such as amounts, due dates, and customer details. Proper clearing control streamlines the cash application process, prevents misallocations, and reduces the risk of errors, enhancing overall financial accuracy and customer satisfaction.
Challenges in Traditional Clearing Control
Traditional clearing control methods in SAP FI-CA/FS-CD rely on fixed rules and preconfigured parameters. While these rule-based approaches work well for straightforward scenarios, they may struggle with more complex situations involving diverse payment methods, partial payments, or ambiguous remittance information. As the volume of transactions increases, the efficiency of rule-based systems may decline, leading to slower processing times and a higher likelihood of manual intervention.
The Role of Machine Learning in Clearing Control
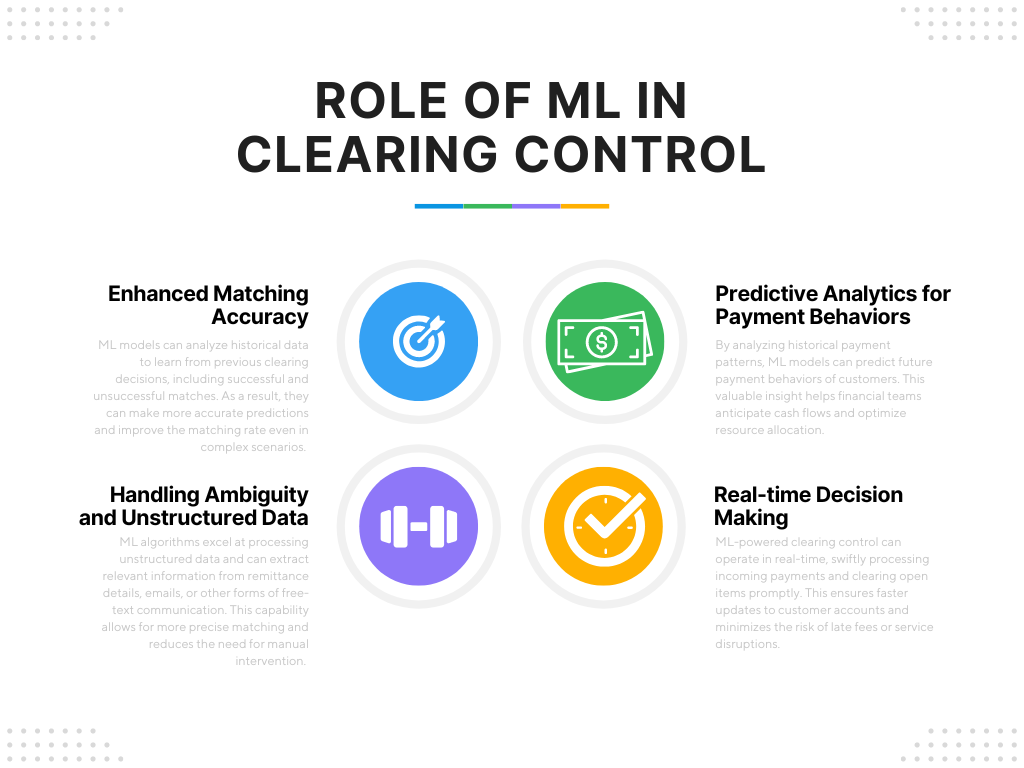
Machine Learning technology revolutionizes the clearing control process in SAP FI-CA/FS-CD by introducing adaptive and data-driven approaches. By leveraging ML algorithms, the clearing control module can make intelligent decisions based on historical data patterns, transaction history, and customer behavior. Below are some key applications of ML in clearing control:
1. Enhanced Matching Accuracy: ML models can analyze historical data to learn from previous clearing decisions, including successful and unsuccessful matches. As a result, they can make more accurate predictions and improve the matching rate even in complex scenarios.
2. Handling Ambiguity and Unstructured Data: ML algorithms excel at processing unstructured data and can extract relevant information from remittance details, emails, or other forms of free-text communication. This capability allows for more precise matching and reduces the need for manual intervention.
3. Real-time Decision Making: ML-powered clearing control can operate in real-time, swiftly processing incoming payments and clearing open items promptly. This ensures faster updates to customer accounts and minimizes the risk of late fees or service disruptions.
4. Predictive Analytics for Payment Behaviors: By analyzing historical payment patterns, ML models can predict future payment behaviors of customers. This valuable insight helps financial teams anticipate cash flows and optimize resource allocation.
Benefits of Machine Learning in Clearing Control
- Improved Efficiency: ML algorithms significantly reduce the need for manual intervention, saving time for finance professionals and increasing overall operational efficiency.
- Higher Accuracy: With ML’s ability to learn from historical data, clearing control accuracy improves, leading to reduced misallocations and fewer exceptions.
- Better Decision Making: ML-driven clearing control empowers organizations to make data-driven decisions, resulting in improved financial planning and strategy.
- Adaptability to Changing Scenarios: Machine Learning models continuously learn from new data, making them adaptable to evolving business requirements and changing payment patterns.
Business Scenario:
While SAP clearing control offers robust flexibility and can be customized with pre-defined business rules to handle complex scenarios for payment applications to open items, there are instances where dealing with intricate situations demands substantial software customization efforts. This customization is based on the available business information at the time, which may not always be sufficient for accommodating the constantly changing payment behaviors in the payment industry.
The need for quick adaptation of the backend to handle the clearing of open items poses challenges in terms of time, effort, and cost. Adding or changing rules/customization in the SAP backend solution becomes an expensive and time-consuming process. Multiple steps need to be executed as part of a change project before the system can automatically handle the evolving business rules for payment assignment.
Firstly, businesses have to manually handle the changing payment behavior, which can be time-consuming and prone to errors. Once the business is confident in the new approach, it must draft its requirements for the change project. Subsequently, the system needs to be configured and tested based on the change request. Only after the business confirms the effectiveness of the changes in the test environment can it be moved to the production environment to handle future transactions automatically.
The tricky part is striking the right balance between the need for speedy adaptation to shifting payment behaviors and minimizing the time, effort, and expense associated with tailoring the SAP backend solution. Businesses must look for alternate options to improve clearance control without making significant software development efforts, such as utilizing machine learning, as the payments sector advances quickly. By adopting machine learning algorithms, businesses can achieve agile and automated payment allocation processes that adapt seamlessly to the constantly changing payment landscape, while reducing the burden of manual customization and project execution. The utilization of machine learning enables both the business and the system to learn concurrently. The ML application can initiate clearing proposals for the business to review and approve in the live environment. As the business gains confidence in the ML-driven approach, it can delegate the responsibility of clearing the application for automated handling.
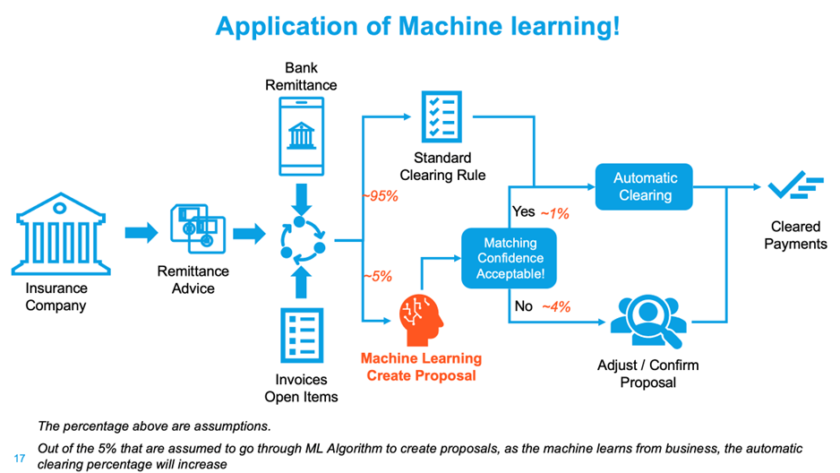
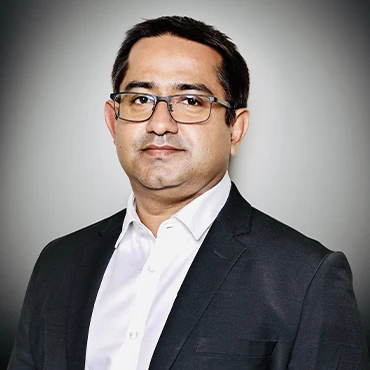
Looking for expertise and excellent know-how in matters of insurance & banking?
Mithlesh Kumar Jha
Managing Partner -SAP FPSL (Insurance & Banking)